Next-Generation Compute Architectures Enabling Artificial Intelligence—Part II of II
In this second of two installments (for Part I, see https://www.futuregrasp.com/next-generation-compute-architectures-enabling-artificial-intelligence-part-I-of-II ), we examine technologies emerging to enable the next generations of commercial AI. Across these exciting approaches, common trends noted in the first wave of AI entrants in the marketplace continue. These include:
·strategies to reduce noise and increase efficiency
·smaller scale engineering
·a proliferation of advanced materials
·increasing speed, density and efficiency
·diversification in mechanism
Nanomaterials
Nanomaterials are considered promising for extending Moore’s Law beyond CMOS [1] as fundamental transistors, as well as copper interconnects connecting the transistors, to enable a whole new class of integrated circuits (ICs). Carbon based nanomaterials, such as carbon nanotubes (CNTs) and graphene, are especially interesting because of their rapid transport of electrons. [2] CNTs can be made in various diameters and chiralities [3] to optimize electron transport for a given application, and may be grown in situ to facilitate fabrication. Graphene can be vapor deposited as a layer and shaped with lithography. [4] Both materials show desirable switching and optical characteristics, as well as good properties for electronic and optical architectures ( vide infra ). [5] On the horizon are materials with even more favorable transport and switching properties, such as MoS2. [6]
Applying nanomaterials such as CNTs can enable whole new chip architectures. Traditional CPUs are two-dimensional (2D) - ICs are produced with numerous layers of valleys, bridges and interconnects on a flat silicon substrate. Multiple ICs are connected on the 2D landscape of a circuit board. Unfortunately, by being 2D the processing speed is inherently limited in CPUs because of the longer time that electrons must take to transport between ICs.
ICs, and thus AI, would benefit with a more 3D architecture. Recent work by MIT and Stanford demonstrates the promise of 3D architectures built on a backbone of millions of CNTs. “As applications analyze increasingly massive volumes of data, the limited rate at which data can be moved between different chips is creating a critical communication ‘bottleneck.’ And with limited real estate on the chip, there is not enough room to place them side-by-side, even as they have been miniaturized.” [7] [8] On-chip storage and processing speed are both increased by sandwiching CNTs between existing silicon chips. This novel approach offers advantages over traditional IC design. “In perspective this chip is important because of its efficiency (low power consumption thanks to the carbon nanotube and fast processing thanks to the colocation of storage and processing) which makes it very interesting in AI applications.” [9]
Of course, a major challenge with incorporating nanomaterials into traditional IC production is to minimize the changes to semiconductor fabs. Hundreds of billions of dollars have been invested over the last 50 years to optimize IC manufacturing with a silicon architecture. Any new material will have to fit within this process flow at minimal cost. At the end of the day, semiconductor production is all about economics - getting the best and most chips manufactured at the lowest cost.
Optical
Another emerging semiconductor technology is optical computing. Instead of electrons, light (photons) is used as the medium to conduct information between transistors. Thus, computations can literally occur at the speed of light, faster than conventional electron-driven chips. Materials enabling optical computing are in general quite different from electronic materials, and materials for optics that can mesh with ASIC and other nanoelectronic fabrication strategies are subject to intensive research. Silicon (and silicon-germanium) have a strong lead for integrated photonic chips; however, its limitations are driving advances in graphene and other materials. [10] [11] [12]
As with any advanced computational architecture, faster processing speed can benefit AI computation. Deep learning especially requires rapid data analysis with large datasets, all while saving energy compared to traditional CPUs or GPUs. Optical chips hold the potential to offload the matrix calculations needed for deep learning to another chip from the CPU, and thereby save power consumption and minimize space use by multiple CPUs. [13] Recently, collaborators from the University of Bristol , Microsoft, Google, Imperial College , Max Planck Institute , and the Sun Yat-sen University invented a new algorithm for solving the energy structure of quantum systems on optical quantum computers. Their device provides an example of quantum algorithm development implemented on an optical device architecture that can yield mass manufacturable systems. [14]
Groups such as at MIT [15] [16] , the University of Arizona [17] , and the University of Alberta in Canada [18] are driving research in advanced optical materials, switches, lasers and nano-optics to further optical computing. For example, a group in the UK recently shrunk the distance a given set of photons must travel on a chip from more than a centimeter to mere micrometers, thus enabling even faster processing speeds. [19] However promising optical chips might be, much research remains for optical computing to enable more calculations on a given chip than nanoelectronics, and as well to increase scalability for full systems capable of AI. However, advances in this space have been advancing in line with other architectures, and we may expect to see greater deployment of light on chips in the future.
DNA and Other Biological Compute Strategies
Just as neuromorphic is a higher level of neural net integration, adapted from observations of biological computing systems, it is no surprise that it is possible to further emulate and co-opt biochemical information processing systems. DNA [20] is the stuff of life, so wouldn’t it be ironic if we were to design a computer that enables AI with the materials from our own genomes? That approach is one of the most exotic in our list of advanced computer architectures that may someday create highly adaptable parallel processing, and high memory storage density ICs.
“DNA computing is a form of parallel computing in that it takes advantage of the many different molecules of DNA to try many different possibilities at once.” [21] [22] One recent claim by the University of Manchester is for a DNA computer that ‘grows’ as it computes, i.e., its compute pathways (the DNA) copy and replicate themselves as a computation is underway to find a given answer faster. [23] This is wholly different from a fixed silicon IC design.
Another major advantage of DNA is in its potential for memory storage. “Capable of storing 215 petabytes (215 million gigabytes) in a single gram of DNA, the system could, in principle, store every bit of datum ever recorded by humans in a container about the size and weight of a couple of pickup trucks.” [24] Microsoft announced in early 2017 that they plan on adding DNA storage to their cloud. [25] While promising, a barrier for such deployment is cost – DNA storage requires both read and write capabilities, which at this writing cost several thousand dollars per megabyte—too expensive for large-scale cloud storage. But as costs come down and read/write speeds and accuracy improve, we may have some of our future social media posts being stored on the very material we depend upon in our cells.
Other molecules have also been adapted for biological compute. [26] RNA and proteins have been used in biochemical/biological computing architectures. [27] Both of these biomolecules interact strongly with each other and their environments to a greater degree than DNA. As they also have programmable structures, a language of RNA and protein shapes that fit like puzzle pieces and interact like gear teeth is ubiquitous for biological information processing. These shapes can form switches and logic gates, and enable massively parallel processing via chemistry held on surfaces and in liquid solution. [28]
One limitation to biological compute (BC) currently, is that, like quantum computing (QC), BC has challenges in manipulating algorithms and in structuring problems that early limited systems can solve. BC has demonstrated real advantages in certain kinds of complex optimization problems also attractive for QC. [29] [30] A few intrepid startups are already active in this space; for example, Rebel Bio recently demonstrated a DNA-based biological computer that plays Tetris. [31] With increased investment in this area, it is anticipated that systems will continue to improve in generality, flexibility, and convenience. [32]
Integration of BC from bacterial systems has been well established. [33] Other species including slime molds harnessed to solve complex problems and integrate environmental sensing. [34] [35] [36] Far beyond simple organisms, ambitious progress has been made interfacing the nanoelectronic approaches with the biological, culminating in the development of AI implanted in a prosthetic to aid human cognition, as being commercialized in a partnership among INTENT LTD , Intel, and Qualcomm. [37] While BC is perhaps the most forward-leaning AI embodiment, it presents a unique challenge as it is the origin of human consciousness. [38]
Conclusions
We emphasized in Part I that “There is now no longer a single Moore’s Law, but rather a suite of them, with each new law a function of compute architecture, software and application.” The additional emerging technologies discussed here in Part II further embrace and extend the portent of this observation. [39]
Diversification in device and integration of devices offer technology options that enable niche applications. As these new capabilities are proven successful, some niches will expand into more general applications. [40] These developments set the stage for waves of M&A [41] as smaller firms are absorbed into larger concerns producing higher volume.
Ultimately, new compute architectures will ensure many additional generations of continuous improvement, well beyond Moore’s Law. AI will thus be driven to ever higher levels of compute and more capable performance.
Notes
[1] Complementary metal-oxide semiconductor
[2] Z. Chen, et al., “Carbon nanotubes for high-performance logic,” MRS Bulletin, 39, August 2014, 719-726.
[3] CNTs come in multiple sizes, including single-walled and multi-walled, as well as chiralities, or ‘handedness,’ such as zigzag and armchair. Such diverse CNT species are tailored toward the desired electrical and other properties. [ https://en.wikipedia.org/wiki/Carbon_nanotube , accessed January 2018.]
[4] https://www.intechopen.com/books/graphene-materials-advanced-applications/fundamentals-of-chemical-vapor-deposited-graphene-and-emerging-applications , accessed January 2018.
[5] http://aip.scitation.org/doi/abs/10.1063/1.4795332?journalCode=apl , accessed January 2018.
[6] https://www.sciencedirect.com/science/article/pii/S1369702115003776 , accessed January 2018.
[7] http://news.mit.edu/2017/new-3-d-chip-combines-computing-and-data-storage-0705 , accessed January 2018.
[8] http://sites.ieee.org/futuredirections/2017/07/10/carbon-nanotubes-powering-the-chip-of-the-future/ , accessed January 2018.
[9] Ibid.
[10] http://iopscience.iop.org/article/10.1088/2040-8978/18/7/073003/meta , accessed January 2018.
[11] http://ieeexplore.ieee.org/document/7409809/ , accessed January 2018.
[12] https://web.stanford.edu/group/heinz/publications/Pub212.pdf , accessed January 2018.
[13] https://www.scientificamerican.com/article/light-powered-computers-brighten-ai-rsquo-s-future/ , accessed January 2018.
[14] http://advances.sciencemag.org/content/4/1/eaap9646 , accessed January 2018.
[15] http://newsoffice.mit.edu/2013/computing-with-light-0704 , accessed January 2018.
[16] https://www.nature.com/articles/nphoton.2017.93 , accessed January 2018.
[17] http://www.ece.arizona.edu/ua-leads-multi-university-team-optical-computing-research , accessed January 2018.
[18] http://www.wirelessdesignmag.com/news/2014/08/electrical-engineers-take-major-step-toward-photonic-circuits?et_cid=4108958&et_rid=281046610&location=top , accessed January 2018.
[19] https://www.sciencedaily.com/releases/2017/11/171130141048.htm , accessed January 2018.
[20] Deoxyribonucleic acid
[21] https://en.wikipedia.org/wiki/DNA_computing#cite_note-16 , accessed January 2018.
[22] Lewin, D. I. (2002). "DNA computing". Computing in Science & Engineering. 4 (3): 5–8. doi : 10.1109/5992.998634 , accessed January 2018..
[23] http://rsif.royalsocietypublishing.org/content/14/128/20160990 , accessed January 2018.
[24] http://www.sciencemag.org/news/2017/03/dna-could-store-all-worlds-data-one-room , accessed January 2018.
[25] https://www.technologyreview.com/s/607880/microsoft-has-a-plan-to-add-dna-data-storage-to-its-cloud/ , accessed January 2018.
[26] https://books.google.com/books?hl=en&lr=&id=fKNJvbRwrY8C&oi=fnd&pg=PA179&ots=_VrR2ZCW-k&sig=wjTl7gnFvFhov8-7uvuvLoYP-oY#v=onepage&q&f=false , accessed January 2018.
[27] https://biodesign.asu.edu/news/living-computers-rna-circuits-transform-cells-nanodevices , accessed January 2018.
[28] http://www.cbc.ca/news/mcgill-bio-super-computer-1.3466095 ?, accessed January 2018.
[29] https://link.springer.com/article/10.3103/S0146411617050108 , accessed January 2018.
[30] https://www.lunduniversity.lu.se/article/using-nanotechnology-to-create-parallel-computers , accessed January 2018.
[31] https://rebelbio.co/fancy-playing-tetris-bio-computer/ , accessed January 2018.
[32] https://blogs.wsj.com/venturecapital/2015/11/18/andreessen-horowitz-launches-200m-bio-computer-fund-hires-ex-stanford-prof/ , accessed January 2018.
[33] https://www.ncbi.nlm.nih.gov/pmc/articles/PMC5264507/ , accessed January 2018.
[34] https://www.sciencedaily.com/releases/2014/03/140327100335.htm , accessed January 2018.
[35] https://phys.org/news/2011-09-biological-cancer-cells.html , accessed January 2018.
[36] https://www.technologyreview.com/s/512821/shrinking-blob-computes-traveling-salesman-solutions/ , accessed January 2018.
[37] https://www.nds.ox.ac.uk/news/researchers-take-major-step-forward-in-artificial-intelligence , accessed January 2018.
[38] http://ubiquity.acm.org/article.cfm?id=1103047 , accessed January 2018.
[39] In 2015, one author [TAC] ran a workshop titled “Moore’s Law 2.0.” One conclusion from the series of presentations by industry and academia is that we now have such a diverse realm of compute architectures that there is confusion in both industry and the US Government on which approach to fund the most. No one knows which chipset will be the winner, if there even will be a clear winner.
[40] https://www.cbinsights.com/research/top-acquirers-ai-startups-ma-timeline/ , accessed January 2018.
[41] Mergers & Acquisitions
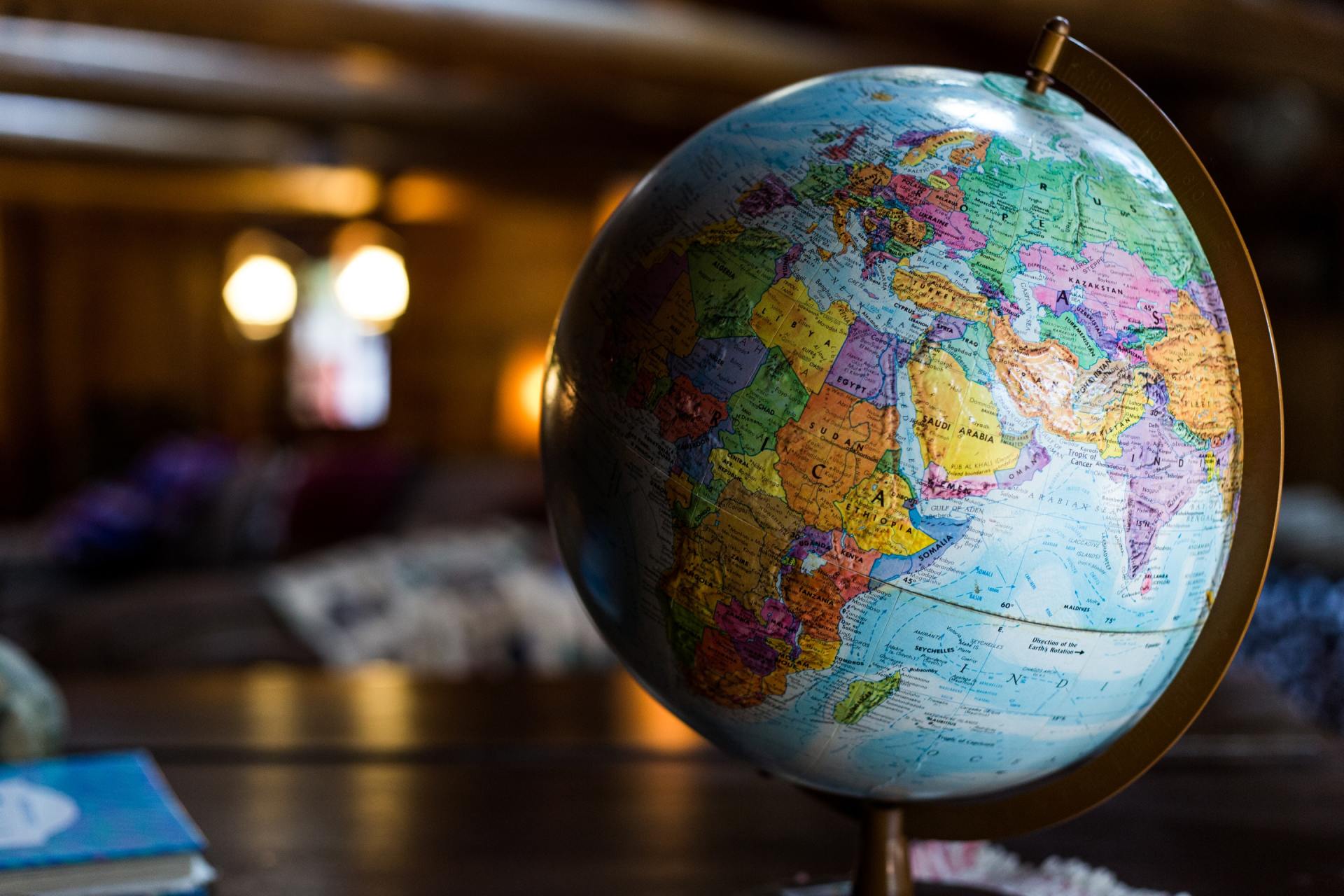
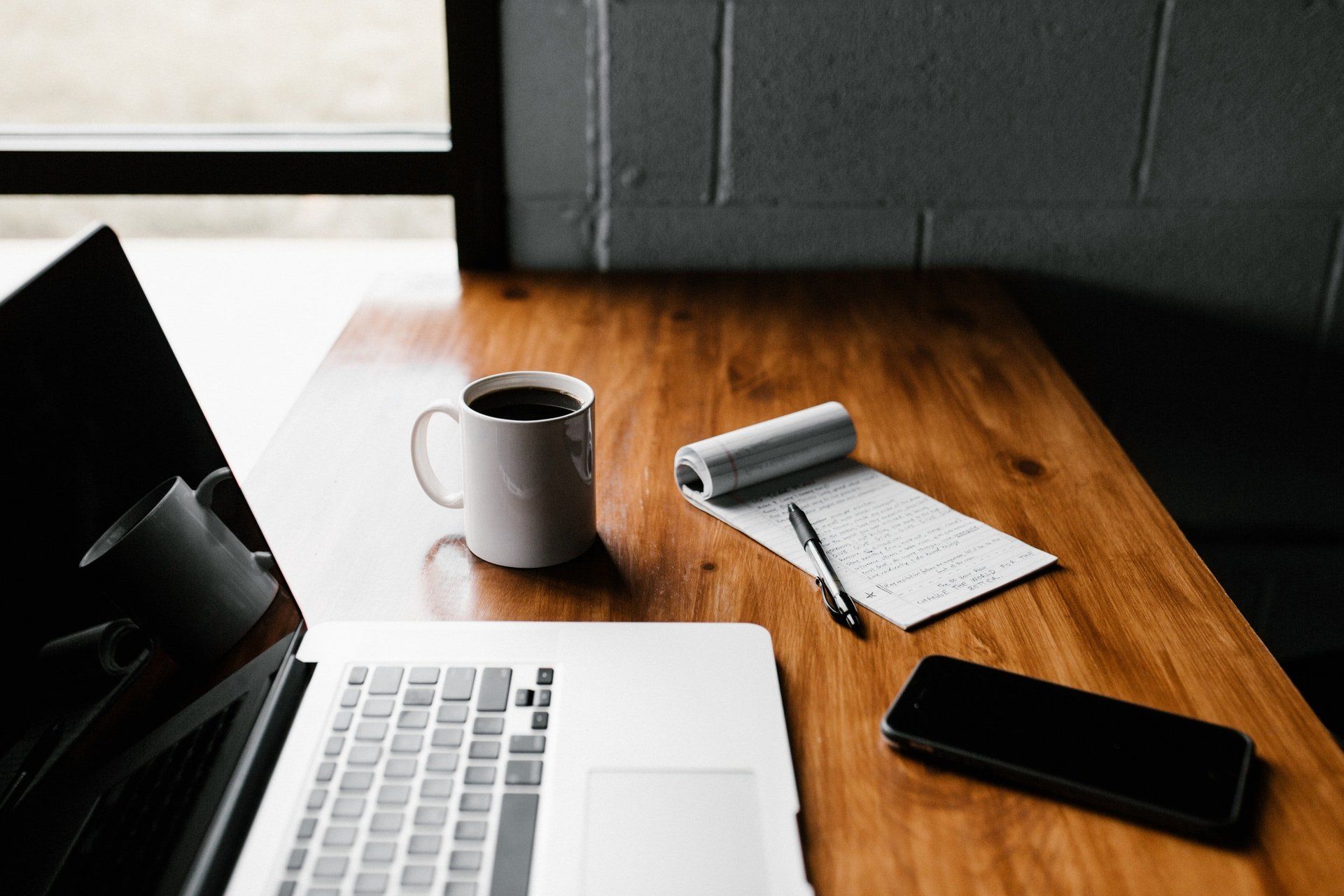
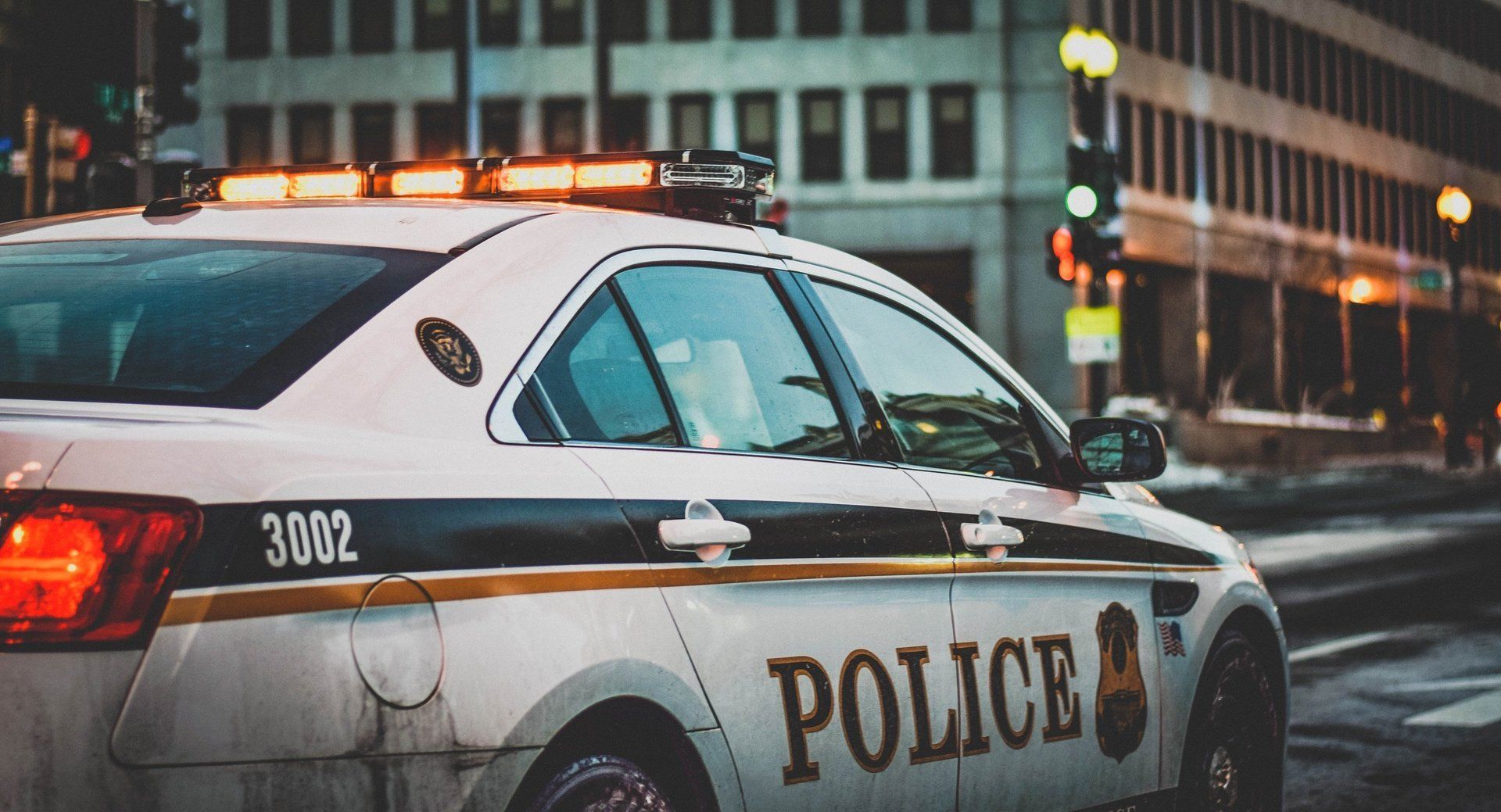
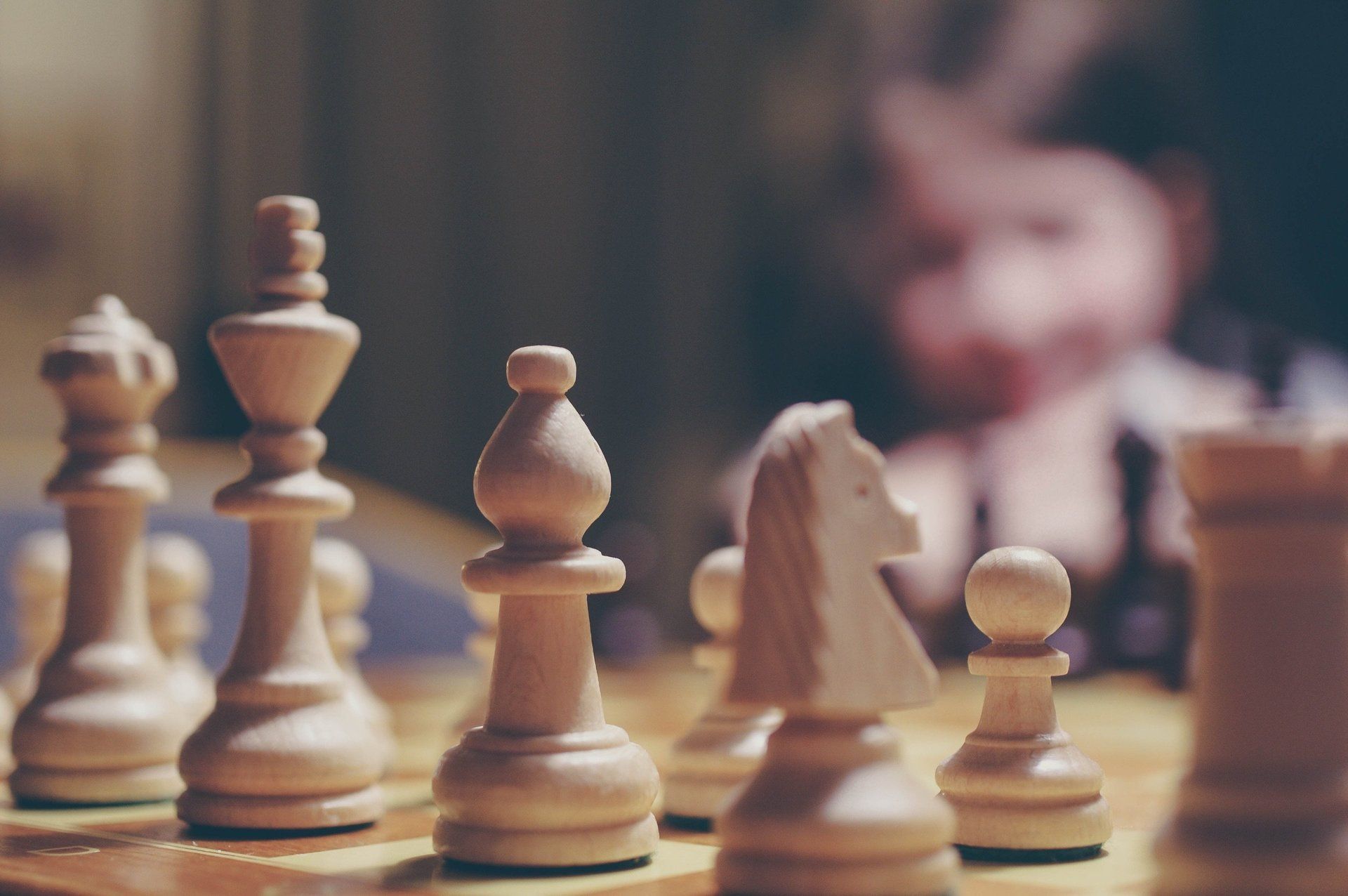