Identifying the Next Big Thing: Qual vs. Quant
Throughout history, humans have remained obsessed with mechanisms for predicting the future. From oracles and auguries of the ancients, to modern day algorithms of Wall Street, we have trusted in diverse tools to foretell what might come next. Today, the union of technology and access to information may enable an unprecedented ability to quantitatively forecast the Next Big Thing in science and technology. However, qualitative tools—despite their many shortcomings—still dominate the domain.
In today’s fast-paced digital economy, a rapid and accurate prediction of science and technology (S&T) – “What will be the Next Big Thing?” – would be a powerful lever for investments, research and defense planning. One frequently sees lists of “Top 10 Technologies.” But for such a quantitative number, such lists are generated generally using qualitative approaches. Experts are convened and hold a meeting (a.k.a., a BOGSAT—bunch of guys sitting around a table), surveys are sent out on the internet to get the crowd’s opinion, and results are pooled into the technologies that supposedly will dominate in the coming year. While certainly offering some level of insight, such qualitative approaches should be recognized for what they are: inherently limited.
CHALLENGES TO QUALITATIVE APPROACHES
Several challenges exist with a purely qualitative approach for S&T analysis.
Information Overload. Even if one gathers hundreds of experts in a scientific discipline and accumulates thousands of votes on the internet, it will still be impossible to truly absorb all the information in S&T, or even in a given field. The amount of information produced globally is now tremendous: every day we create 2.5 quintillion bytes of new data [1] , and every year there are roughly 2.5 million journal articles published [as assessed from a 2014 study]. [2] No one expert, or even group of experts, can absorb all this information, contextualize it, and make substantive predictions from it.
Specialization. As a ‘new’ field becomes more mainstream and grows, it tends to splinter into specialty fields, thus challenging anyone to remain a comprehensive expert. For example, 3D-printing used to be a little-known subset of mechanical engineering. Capabilities were limited primarily to cheap plastics and a handful of commercial printers. But as more researchers got involved and advances were made, the availability to 3D-print exploded into hundreds of materials and systems. Now almost any material, including limited types of food and organs, can be 3D-printed. If one was considered an expert in the early days of 3D-printing and able to answer any question on it, it became very difficult, if not impossible, to do so as the field expanded. [3] Nowadays, experts are specialized in niche areas across most S&T disciplines – e.g., nanotechnology is now splintered into carbon nanotech, quantum dots, bio-nanotech, etc. In short, in the nascent days of an S&T space, one might realistically claim to be an omniscient expert, whereas as the field matures one cannot. The BOGSAT requires many more people and a really big table as a field expands to possess all-encompassing expertise.
Convergences. S&T fields tend to influence each other in unexpected ways. One could posit that the low-hanging fruit in silo’d S&T specialties has already been plucked, and that to get to the high-hanging fruit one must get a ladder that can converge disparate S&T branches together. Take, for example, artificial intelligence (AI), specifically deep learning. In repeated cycles of hype and disappointment, AI went through two or more ‘winters’ in which funding dried up. In the early days of AI, a core limiting aspect was computational power. Most recently, researchers have leveraged graphics processing units (GPUs), which were originally designed for gaming, to execute the massive computations required for deep learning. By doing so, AI has accelerated well beyond its origins from Alan Turing in the 1950s. Thus, gaming chips enabled AI, which enabled new approaches to computation, and did so in an unexpected manner.
Emergences. New discoveries are often the result of serendipity, where chance favors the prepared mind. However, the germination of a new discovery or invention may be completely missed, no matter how much of an expert one is. Much of research is unpublished. A lab book note, a university seminar, a small workshop – these are the only inklings one might encounter that something new is on the cusp of being debuted. In their excellent book, A Crack in Creation: Gene Editing and the Unthinkable Power to Control Evolution, authors Doudna and Sternberg [4] detail the earliest days of CRISPR [5] research that presaged the incredible levels of investment and excitement now prominent in the biotechnology community. Only a handful of researchers were involved in the early stages of CRISPR. Unless one is truly in the know , such knowledge is missed until it goes mainstream.
Non-Technical Influencers. Context is important and never more so than today where data, information (and disinformation), and judgements are readily shared and propagated globally. Consequently, the perception of S&T held by its developers, financiers, customers, and even the general public can greatly influence the evolution and acceptance of an innovation. For example, the view of a technology such as genetically modified organisms, GMOs, varies with the culture and economy of different regions of the world, and changes temporally as the technology matures, is marketed, and its perceived successes and failures are shared. Thus, it has become critical to consider non-technical influences, such as market dynamics, when assessing the S&T outlook. Such factors and relationships are far too complex for an individual or team to interpret.
FROM QUAL TO QUANT
So what can we do to better grapple with the exponentially growing S&T space? How can we identify the subtle ripples in the S&T community that might signal a profound discovery? Can we begin to understand the impact of the non-science community on the financing, development and acceptance of S&T and its products and services derivatives?
To contend with these issues, we should leverage a more quantitative approach to identifying, tracking, forecasting and assessing S&T. Humans should still remain in the loop, but our abilities can be augmented beyond our limited organic framework. We often have a hard time exchanging information among ourselves (we can all recall death-by-PowerPoint meetings); we must sleep and eat; and we enjoy time for entertainment, family and friends. Computers are excellent networkers and need no such organic supports.
There is a wealth of open source information available for the properly coded algorithm. The figure above shows several examples of technical influencers upon S&T knowledge. There are three main technical sources of information on S&T: the internet, publications and meetings. Although not claiming to be comprehensive, multiple examples of each are listed in the sidebar tables. Within each row, one might also consider additional information such as authors, citations and number and origins of people attending meetings. To construct a comprehensive dataset on even a sub-field within S&T would require substantial compute and memory storage.
Ultimately, the ability to make meaning of such data is hampered by many factors. An unproven algorithm, disparate data, lack of data, misinformation, fake news - all can obfuscate and compromise a prediction’s accuracy, or even its proximity to any reality at all. Such issues must be considered to produce a rigorous quantitative tool. To the authors’ knowledge, no organization has been able to fully leverage all the available information we now possess on S&T with a quantitative approach. It is certainly worthwhile to develop such a program.
A PRESAGE APPROACH
In 2011, the Intelligence Advanced Research Projects Activity (IARPA) created the Open Source Indicators (OSI) program that challenged technology developers to create a capability that harvests open source datasets to make predictions, or otherwise forecast significant societal events before they occur. [6] The leading technology and winner of the OSI challenge, now offered by VT-ARC commercially as PreSage, was developed by Virginia Tech and serves as a base platform for several other predictive analytics challenges. Could such a tool provide the quantitative means to overcome the challenges faced by qualitative approaches to S&T analytics?
PreSage—or other automated systems of big data collection, fusion and analysis employing natural language processing (NLP), topic analysis, clustering, and other machine learning techniques—has the potential to significantly improve S&T analytics by augmenting the human analyst’s work and providing quantitative insights where previously qualitative approaches dominated. For example, an automated, AI-driven tool could:
·Rapidly ingest and process very large volumes of data in near real-time thereby eliminating the information overload encountered by analysts tracking S&T,
·Track and maintain a deep understanding of technical fields even as they become more specialized ,
·Identify capabilities and shortfalls from seemingly unlinked S&T domains where convergence can create opportunity,
·Detect early indicators of S&T emergence such that decisions can be made proactively and with confidence, and
·Construct a holistic view of S&T inclusive of non-technical information that nevertheless shapes the evolution and progression of technology.
PreSage has already demonstrated the ability to ingest and process a broad range of publicly available data such as that shown in the figure; track and maintain large volumes of data, including streaming data; and fuse disparate datasets into a cohesive prediction. What’s necessary next is the development of appropriate models to describe the variables and relationships specific to S&T, integrate them with non-technical indicators, and identify training and test datasets—a non-trivial, yet achievable challenge. VT-ARC and FutureGrasp seek partners in this exciting journey to construct quantitative models for better forecasting and assessments of S&T.
CONCLUSIONS
What then, is to come? If a successful S&T prediction program is realized, how might it be used to assist the scientist? Influence the investor? Inform the marketer? Defeat the adversary?
As the power and utility of AI-driven products and services continue to grow rapidly, AI itself should be leveraged to quantitatively forecast the progression of S&T more broadly. The predictive nature of tools such as PreSage offers to provide not only greater insight into which technologies will deliver greater capability in years to come, but also those that will incite investment and consumer interest, and lead to commercial success.
Ultimately, the production of information will never cease growing. Decisions need to be made more expediently and with greater rigor. A more quantitative approach toward S&T analysis would benefit anyone interested in trying to identify the Next Big Thing.
ACKNOWLEDGEMENTS
The authors gratefully acknowledge Luke Sebby of VT-ARC for his review of this note.
NOTES
[1] https://www-01.ibm.com/common/ssi/cgi-bin/ssialias?htmlfid=WRL12345USEN , accessed January 2018.
[2] http://www.stm-assoc.org/2015_02_20_STM_Report_2015.pdf , accessed January 2018.
[3] One author [TAC] can attest to this first-hand, as he researched 3D-printing at Virginia Tech from 2007 to 2013.
[4] A Crack in Creation: Gene Editing and the Unthinkable Power to Control Evolution, Jennifer A. Doudna, Samuel H. Sternberg, 2017, Houghton Mifflin Harcourt Publishing Company.
[5] CRISPR is the acronym for “Clustered Regularly Interspaced Short Palindromic Repeats,” which refers to short segments of DNA, the molecule that carries genetic instructions for all living organisms. A few years ago, the discovery was made that one can apply CRISPR with a set of enzymes that accelerate or catalyze chemical reactions in order to modify specific DNA sequences. This capability is revolutionizing biological research, accelerating the rate at which biotech applications are developed to address medical, health, industrial, environmental, and agricultural challenges, while also posing significant ethical and security questions.
[6] The technology described, developed by Prof. Naren Ramakrishnan of Virginia Tech, utilizes open source data including tweets, Facebook pages, news articles, blog posts, Google search volume, Wikipedia, meteorological data, economic and financial indicators, coded event data, online restaurant reservations (OpenTable), and satellite imagery. Called EMBERS, the technology successfully forecasted events such as the “Brazilian Spring” (June 2013), Hantavirus outbreaks in Argentina and Chile (2013), and violent protests led by Venezuelan students (Feb 2014). The EMBERS technology has since successfully transitioned to the US Government.
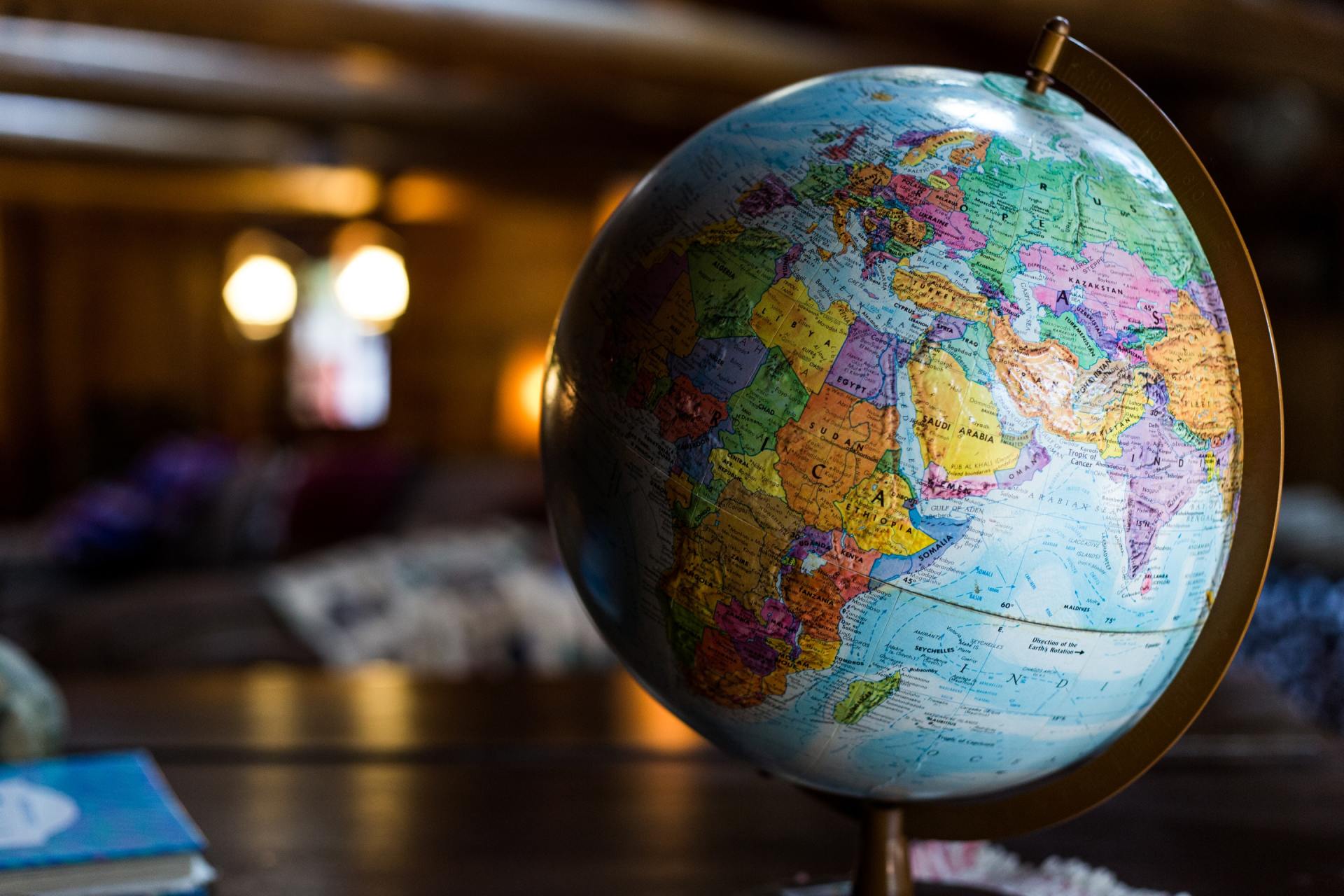
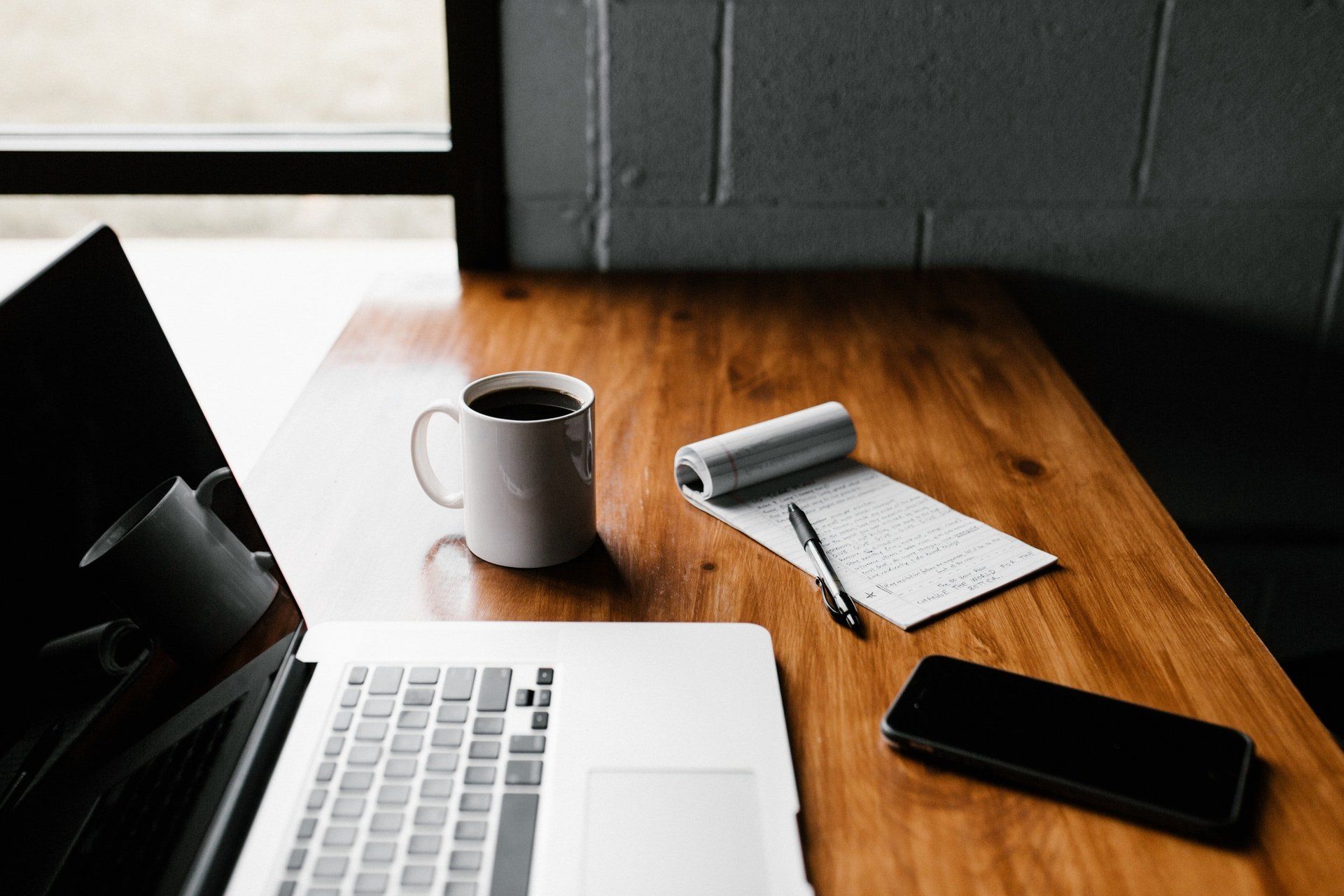
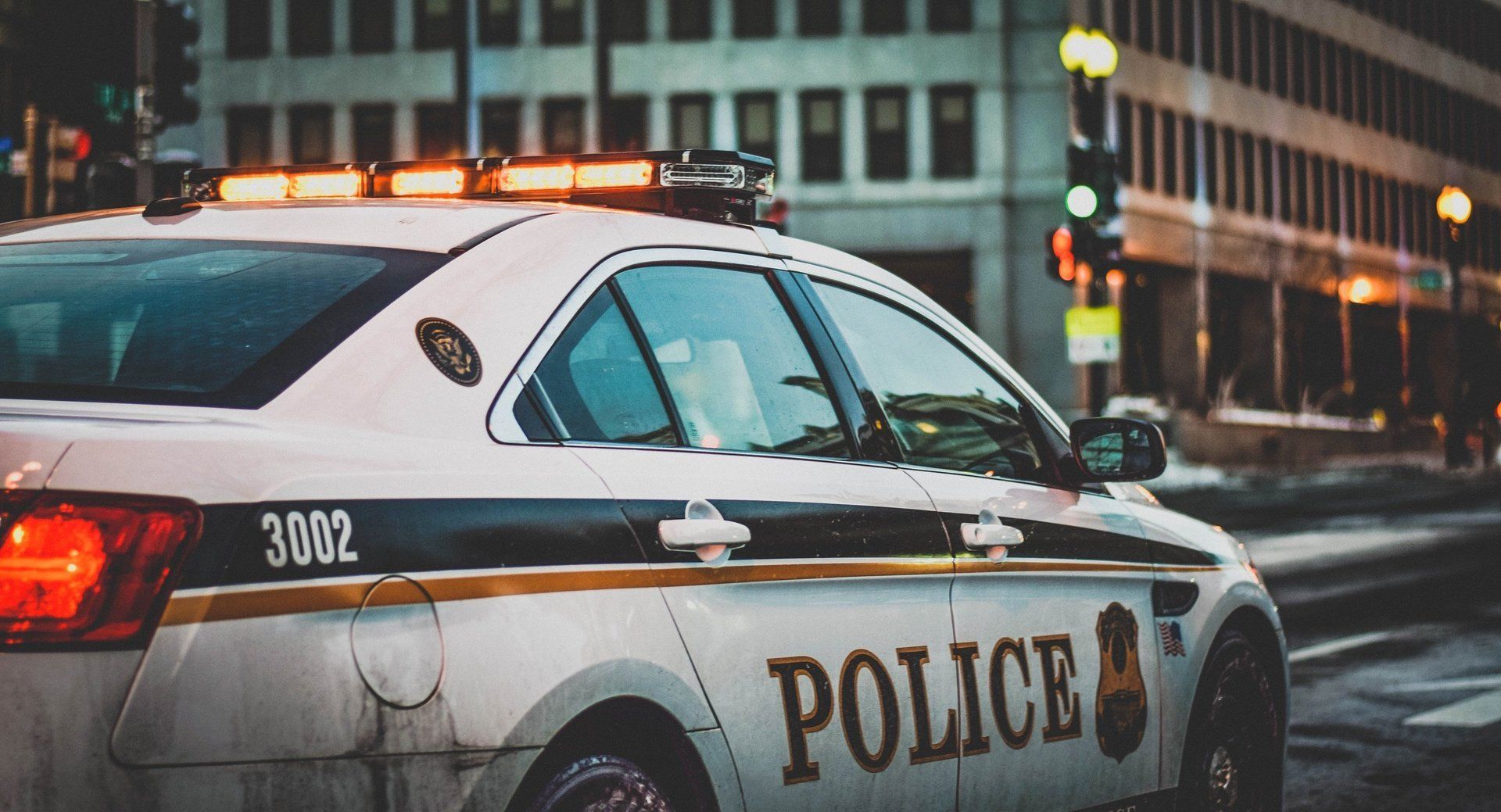
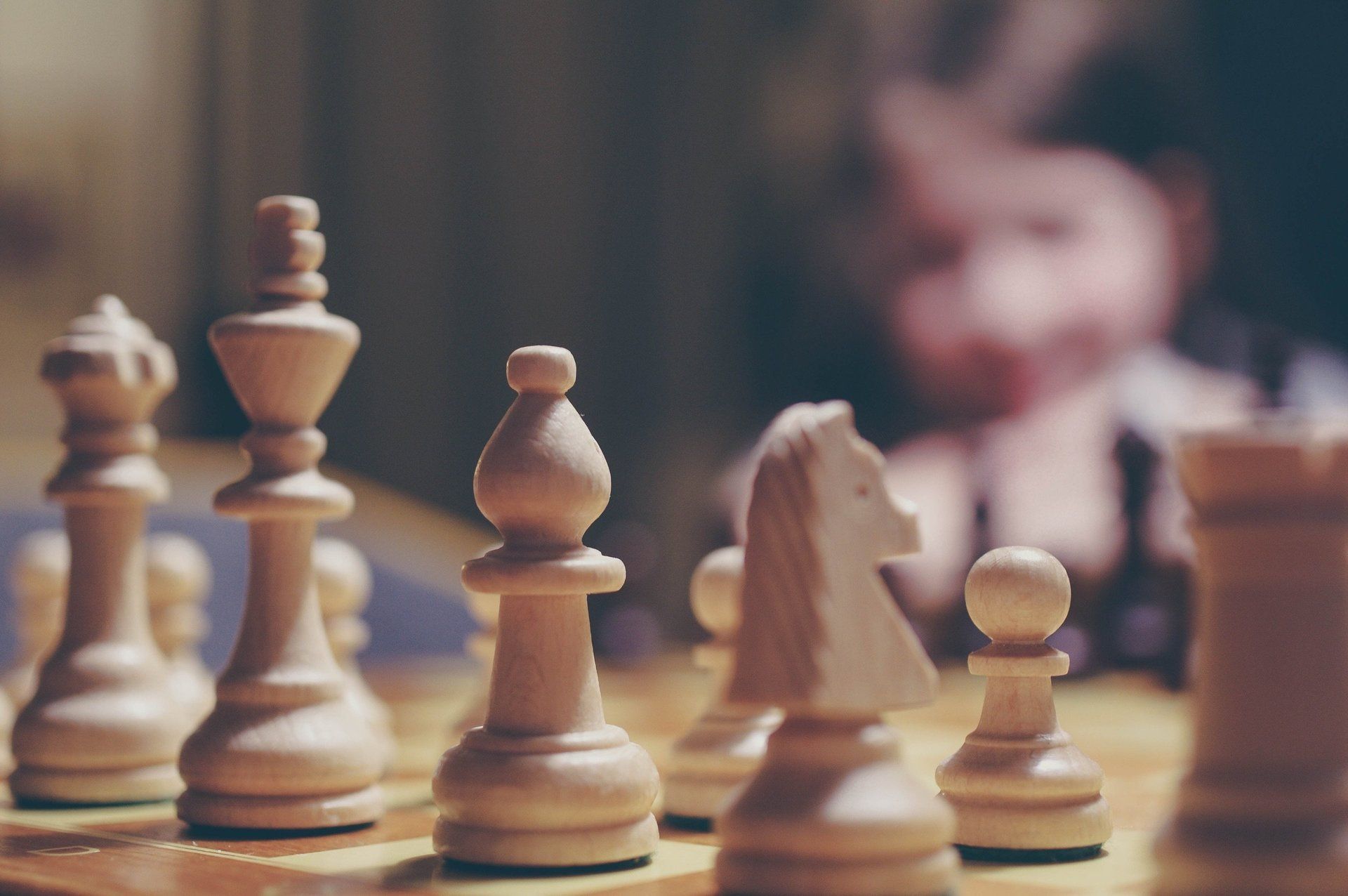