Next-Generation Compute Architectures Enabling Artificial Intelligence - Part I of II
In this first of two installments, we explore the emergence and impact of compute architectures behind artificial intelligence (AI) now in the marketplace. In Part II (see https://www.futuregrasp.com/next-generation-compute-architectures-enabling-artificial-intelligence-p... ) we look at emerging compute technologies that might influence the next generations of commercial AI.
Death of Moore’s Law
In 1965, Dr. Gordon E. Moore wrote an article based on a trend he noticed: the number of transistors in an integrated circuit (IC) doubles approximately every two years [1]. Fueled by unrelenting demands from more complex software, faster games and broadband video, Moore’s Law has held true for over 50 years. [2] It became the de-facto roadmap against which the semiconductor industry drove its R&D and chip production, as facilitated by SEMATECH’s pre-competitive agreements among global semiconductor manufacturers. Recently, that roadmap has faltered due to physics limitations and the high cost-benefit economics incurred by the incredibly small scales that chip manufacturing has reached. Electron leakages and difficulties shaping matter at the single-digit nanometer scales of the transistors fundamentally limit further miniaturization. So many electrons are being moved though such tight spaces so quickly that there is an entire field in the semiconductor industry devoted just to chip cooling; without thermal controls, the ICs simply fry and fail. A new fabrication plant (fab) can cost more than $10 billion, severely limiting the number of companies able to produce denser ICs.
Despite the looming end of Moore’s Law, computationally-intensive artificial intelligence (AI) has exploded in capabilities in the last few years – but how, if compute is slowing down? The solution to exceeding compute limitations of traditional von Neumann style central processing units (CPUs) [3] has been to invent and leverage wholly new architectures not dependent on such linear designs [4].
A veritable zoo of compute architectures – including GPUs [5] , ASICs [6] , FPGAs [7] , quantum computers, neuromorphic chips, nanomaterial-based chips, optical-based ICs, and even biochemical architectures - are being researched and/or implemented to better enable deep learning and other instantiations of AI. Here we review the latest / greatest [8] of non-CPU computer architectures relevant to AI. In each section, we describe the hardware, its impact to AI, and a selection of companies and teams active in its R&D and commercialization.
GPU
Graphics processing units (GPUs) have taken the computing world by storm in the last few years. Fundamentally, GPUs operate differently than CPUs. “A simple way to understand the difference between a GPU and a CPU is to compare how they process tasks. A CPU consists of a few cores optimized for sequential serial processing, while a GPU has a massively parallel architecture consisting of thousands of smaller, more efficient cores designed for handling multiple tasks simultaneously.” [9]
As deep learning requires high levels of computational power, AI is a particularly strong application for GPUs. Neural network implementation on GPU-based systems accelerates the training process dramatically compared to traditional CPU architecture; this saves substantial time and money. [10]
Nvidia is one of the earliest companies to apply GPUs to AI; its latest TITAN V PC GPU is designed specifically for deep learning in a desktop platform. [11] A US-based company, Nvidia is now a powerhouse that in Q3, 2017 controlled 72.8% of the discrete GPU market share. [12] As more OEMs [13] invest in R&D, we can expect continuous improvements in the rapidly advancing space of GPUs.
ASIC
An application-specific integrated circuit (ASIC) is an IC customized for a particular use, rather than intended for general-purpose use. [14] The advantage for AI is that an ASIC can be designed explicitly for fast processing speed and/or massive data analysis via deep learning. While ASIC processors typically implement traditional von Neumann architecture, foundry-produced ASIC chips with neural net architecture have found niche applications commercially.
There have been several press releases about companies going their own route with ASICs to become independent of general GPUs. [15] Realizing their loss of market share and wishing to not be wholly dependent upon Nvidia, numerous software firms and OEMs are designing and/or implementing their own ASICs. [16] Google is already on its second version of its TPU (tensor processing unit), designed to accelerate processing speeds in their vast server farms. [17] Intel has been working on its own AI-dedicated chip for several months. [18] Intel claims its Nervana Neural Network Processor family, or NNP for short, is on track to increase deep learning processing speeds by 100 times by 2020. [19] Tesla recently announced its efforts to build from scratch its own AI chips to have better control and faster processing speed in its electric cars. [20]
FPGA
A field-programmable gate array (FPGA) enables greater customization after manufacturing—ergo, field-programmable . “The FPGA configuration is generally specified using a hardware description language (HDL), similar to that used for an application-specific integrated circuit (ASIC). FPGAs contain an array of programmable logic blocks, and a hierarchy of reconfigurable interconnects that allow the blocks to be ‘wired together’, like many logic gates that can be inter-wired in different configurations.” [21]
While somewhat larger in scale than analogous ASIC processors, a key advantage offered by FPGAs for AI is in their application of deep neural networks requiring analysis of large amounts of data. The flexibility of programming circuitry rather than instructions enables complex neural nets to be configured and reconfigured seamlessly in a standardizable platform. [22] Intel applies FPGAs toward such deep data problems. [23] [24] Their FPGAs were shown to be competitive to GPUs earlier this year:
“While AI and DNN research favors using GPUs, we found that there is a perfect fit between the application domain and Intel’s next generation FPGA architecture. We looked at upcoming FPGA technology advances, the rapid pace of innovation in DNN [deep neural network] algorithms, and considered whether future high-performance FPGAs will outperform GPUs for next-generation DNNs. Our research found that FPGA performs very well in DNN research and can be applicable in research areas such as AI, big data or machine learning which requires analyzing large amounts of data. The tested Intel Stratix 10 FPGA outperforms the GPU when using pruned or compact data types versus full 32 bit floating point data (FP32). In addition to performance, FPGAs are powerful because they are adaptable and make it easy to implement changes by reusing an existing chip which lets a team go from an idea to prototype in six months—versus 18 months to build an ASIC.” [25] [26]
Beyond the traditional semiconductor OEMs, numerous software companies are developing FPGAs to enable faster processing speeds within their data centers. In August 2017, Amazon , Baidu and Microsoft announced manufacturing of FPGAs targeted toward machine learning. “Taken together, these initiatives point to increased opportunities for other large AI consumers and providers to have their cake and eat it too; they can optimize custom chips for their applications while avoiding the cost and potential technology obsolescence of going down the custom ASIC approach.” [27]
Quantum
Traditional computers operate with bits that are either ‘on’ or ‘off’ depending on their electrical state; quantum computers work differently. Quantum computers use qubits , in which an individual bit can be in one of three states: on, off, and, uniquely, both on and off simultaneously. As with other advanced computational architectures, quantum computing (QC) is still in early stages of development. QC has unique hardware issues – for example, the need to cool the processing chip to fractions of a single digit Kelvin to avoid disturbing processing in the qubits. QC also requires a wholly new approach to software since the binary on/off paradigm is no more, and instructions do not load sequentially, but rather execute simultaneously , from which the speed originates. Potential areas where QC could have significant impact include energy (by accelerating discovery in high energy plasma manipulation, materials chemistry, and other optimization modeling problems), medicine (by modeling complex biochemical systems to accelerate drug discovery and understanding of disease), information (by enabling big data analytics and new encryption approaches), aerospace (by modeling hypersonic aerodynamics), and materials (by simulating complex materials systems).
Speed is the leverage that QC offers AI, especially in machine learning. QC has the potential to spot patterns extremely quickly within large data sets. “It might be possible for the quantum computers to access all items in your database at the same time to identify similarities in seconds.” [28] For an AI that must analyze billions of images or data points, to execute this analysis in seconds using the power of the simultaneous on-off qubit is huge compared to a sequential CPU that might take weeks or months for the same action.
Critical challenges to all QC strategies include reducing noise and maintaining system coherence. Improved materials and architectures will continue to drive QC development. [29]
The Canadian company D-Wave Systems is one of the leaders in QC. Although there is some controversy on whether D-Wave actually makes quantum computers (their systems are marketed as more limited quantum annealers [30] [31] ), their multi-million dollar systems have been purchased by Google , Lockheed Martin and others in the past few years. D-Wave recently installed their latest 2000Q System at NASA Ames. [32] Because it is so different than traditional computing, a core challenge with QC is the dearth of software dedicated to operating in both qubit states (on and off) simultaneously. D-Wave partnered with 1QBit to meet this challenge; for example, initial solutions of previously intractable protein folding problems are being explored by 1QBit using D-Wave’s systems.
Several other companies are active in hardware and/or software development for QC. IBM announced recently they reached a threshold of 50 qubits in operation; such a system would be the largest quantum computer to date. However, “…as revolutionary as this development is, IBM's 50-qubit machine is still far from a universal quantum computer.” [33] Microsoft also recently released a preview version of its Q# (pronounced “Q sharp”) software dedicated to quantum computing. “Microsoft's hope is that this selection of tools, along with the training material and documentation, will open up quantum computing to more than just physicists.” [34] Microsoft and IBM both teased major advances in QC, recently. [35] Rigetti Computing is a startup focused on QC, with applications to AI. They are building a 19 qubit quantum computer to run clustering algorithms. [36] [37] While much R&D remains to create a practical application of their computer and algorithms, investors are still interested. “The company [Rigetti Computing] has raised around $70 million from investors including Andreessen Horowitz, one of Silicon Valley’s most prominent firms.” [38]
As quantum computers become more capable with the addition of more qubits and customized software, we can expect more AI experts to seek out their unique capabilities. Visionary venture capital firms such as BootstrapLabs have held special conference sessions on the opportunities that QC presents to AI. [39]
Neuromorphic
For all that they can accomplish, traditional computers still do not mimic the human mind. CPUs operate in a sequential manner, and, at best, GPUs operate via parallel processing. The mind, however, works highly nonlinearly. Each of our roughly 87 billion neurons has up to 10,000 connections to other nerve cells, externally, and internally hosts hundreds of thousands of coordinated parallel processes (mediated by millions of protein and nucleic acid molecular machines). We routinely use almost all of our neurons in countless waves of electrical signals to process information, all while consuming only tens of watts (compared to thousands of watts for a standard computer) [40]. A chip mimicking the brain’s architecture has the potential to be both more efficient and consume far less power than even the most advanced present-day systems. Neuromorphic computing is one approach for pursuing these advantages.
In a 2014 publication in Science , IBM described a new, ‘brain-like’ approach to IC design [41]. This represents a dramatic jump in scale and flexibility beyond the small processors featuring neural net-based circuitry that have been integrated within computer vision systems and other pattern recognition-heavy applications. [42] It is also fundamentally different from neural nets implemented within FPGA, since neuromorphic chips are optimized for neural net architecture. “The new chip, called TrueNorth, marks a radical departure in chip design and promises to make computers better able to handle complex tasks such as image and voice recognition-jobs at which conventional chips struggle…Each TrueNorth chip contains 5.4 billion transistors wired into an array of 1 million neurons and 256 million synapses. Efforts are already underway to tile more than a dozen such chips together.” [43] IBM also coded entirely new software and encourages computer scientists to create new applications with their neuromorphic chip. In very recent research, investigators at NIST have integrated superconducting Josephson junctions [44] (featured in the D-Wave quantum annealers) into neuromorphic chips delivering a blistering 1GHZ firing rate using as little as 1 atto-Joule in a 3-dimensional architecture. [45]
Another device, the memristor, has been demonstrated to have advantages in power and speed for neuromorphic architectures. These 70 nanometer wide devices were integrated to provide 10 trillion (1E12) operations per watt (compared with a CMOS [46] benchmark approximately three orders of magnitude smaller), engaged in solving the Traveling Salesman Problem. [47]
Going to the next level, Intel announced in September 2017 a neuromorphic chip designed explicitly for AI. [48] “Intel's Loihi chip has 1,024 artificial neurons, or 130,000 simulated neurons with 130 million possible synaptic connections. That's a bit more complex than, say, a lobster's brain, but a long ways from our 80 billion neurons…By simulating this behavior with the Loihi chip, it can (in theory) speed up machine learning while reducing power requirements by up to 1,000 times. What's more, all the learning can be done on-chip, instead of requiring enormous datasets. If incorporated into a computer, such chips could also learn new things on their own, rather than remaining ignorant of tasks they haven't been taught specifically.” [49]
As with other non-von Neumann architectures, software must be developed from scratch to enable the capabilities of neuromorphic computing. Companies such as Applied Brain Research are developing software such as Nengo and Spaun to simulate the mind, while the military and private companies such as Inilabs , sponsors of the Misha Mahowald Prize for Neuromorpic Engineering, and PegusApps are targeting this software gap as an opportunity in machine vision and acoustic pattern recognition . [50] [51] [52] [53]
It remains to be seen how advantageous mimicking the mind is for AI. Neuromorphic computing shows promise, but major advances are still needed in hardware (to provide more ‘neurons’ and their connections) and compatible software.
Conclusions from Part I
There is now no longer a single Moore’s Law, but rather a suite of them, with each new law a function of compute architecture, software and application. Major impetuses for this semiconductor speciation are the intense power and speed demands from AI. As more hardware and software tools become available to AI researchers, specialization will inevitably occur. GPUs, ASICs and FPGAs lead the pack now in terms of AI commercial applications, but if sufficient advances are made in quantum computers and neuromorphic computers we may see wholly new, currently inconceivable applications of AI. [54] This promise makes it potentially timely to invest early in some of these technologies. [55]
Part II of this series will explore further emerging compute technologies such as nanomaterials, optical computing, and DNA and other biological compute strategies. Such capabilities may influence future generations of commercial AI.
Notes
[1] Gordon E. Moore, "Cramming more components onto integrated circuits" Electronics, pp. 114-117, April 19, 1965.
[2] The 50th anniversary of Moore’s Law was celebrated in 2016.
[3] Central Processing Unit
[4] A “von Neumann design” refers to a traditional, linear compute CPU.
[5] Graphics Processing Unit
[6] Application Specific Integrated Circuit
[7] Field Programmable Gate Array
[8] As of January 2018. The semiconductor field moves so fast that much of what is written here will be outdated in terms of specific chip and other descriptions within weeks of this publication; however, broad concepts should remain relevant. It should also be noted that we don’t attempt to be comprehensive in terms of companies or specific hardware in this memo.
[9] http://www.nvidia.com/object/what-is-gpu-computing.html , accessed January 2018.
[10] https://www.analyticsvidhya.com/blog/2017/05/gpus-necessary-for-deep-learning/ , accessed January 2018.
[11] https://www.cnet.com/news/nvidias-3000-titan-v-gpu-offers-massive-power-for-machine-learning/ , accessed January 2018.
[12] https://www.fool.com/investing/2017/12/06/nvidia-is-running-away-with-the-gpu-market.aspx , accessed January 2018.
[13] Original equipment manufacturers
[14] https://en.wikipedia.org/wiki/Application-specific_integrated_circuit , accessed January 2018.
[15] Technically, a GPU itself is an ASIC designed for gaming calculations, but we won’t split hairs.
[16] https://www.forbes.com/sites/moorinsights/2017/08/04/will-asic-chips-become-the-next-big-thing-in-ai/#61aabc7211d9 , accessed January 2018.
[17] https://www.nextplatform.com/2017/05/17/first-depth-look-googles-new-second-generation-tpu/ , accessed January 2018.
[18] https://www.theverge.com/circuitbreaker/2017/10/17/16488414/intel-ai-chips-nervana-neural-network-processor-nnp , accessed January 2018.
[19] https://newsroom.intel.com/news-releases/intel-ai-day-news-release/ , accessed January 2018.
[20] https://www.theverge.com/2017/12/8/16750560/tesla-custom-ai-chips-hardware , accessed January 2018.
[21] https://en.wikipedia.org/wiki/Field-programmable_gate_array , accessed January 2018.
[22] https://software.intel.com/en-us/articles/machine-learning-on-intel-fpgas , accessed January 2018.
[23] http://www.newelectronics.co.uk/electronics-technology/fpgas-key-to-machine-learning-and-ai-apps/156849/ , accessed January 2018.
[24] https://seekingalpha.com/article/4101617-intel-fpgas-powers-microsofts-real-time-artificial-intelligence-cloud-platform , accessed January 2018.
[25] https://www.nextplatform.com/2017/03/21/can-fpgas-beat-gpus-accelerating-next-generation-deep-learning/ , accessed January 2018.
[26] https://dl.acm.org/citation.cfm?id=3021740 , accessed January 2018.
[27] https://www.forbes.com/sites/moorinsights/2017/08/28/microsoft-fpga-wins-versus-google-tpus-for-ai/#daa964939045 , accessed January 2018.
[28] https://www.forbes.com/sites/bernardmarr/2017/09/05/how-quantum-computers-will-revolutionize-artificial-intelligence-machine-learning-and-big-data/#2d0bf8df5609 , accessed January 2018.
[29] https://www.quantamagazine.org/the-era-of-quantum-computing-is-here-outlook-cloudy-20180124/ , accessed January 2018.
[30] “Quantum annealing is a metaheuristic for finding the global minimum of a given objective function over a given set of candidate solutions (candidate states), by a process using quantum fluctuations.” https://en.wikipedia.org/wiki/Quantum_annealing , accessed January 2018.
[31] https://blogs.scientificamerican.com/guest-blog/is-it-quantum-computing-or-not/ , accessed January 2018.
[32] https://www.dwavesys.com/press-releases/d-wave-2000q-system-be-installed-quantum-artificial-intelligence-lab-run-google-nasa , accessed January 2018.
[33] https://futurism.com/ibm-announced-50-qubit-quantum-computer/ , accessed January 2018.
[34] https://arstechnica.com/gadgets/2017/12/microsofts-q-quantum-programming-language-out-now-in-preview/?utm_source=Daily+Email&utm_campaign=bfc784b865-EMAIL_CAMPAIGN_2017_12_12&utm_medium=email&utm_term=0_03a4a88021-bfc784b865-248705265 , accessed January 2018.
[35] https://www.ft.com/stream/ab49fad4-07f5-3950-9aa0-87cdd74ce0b8 , accessed January 2018.
[36] https://www.technologyreview.com/s/609804/a-startup-uses-quantum-computing-to-boost-machine-learning/?utm_source=twitter.com&utm_medium=social&utm_content=2017-12-18&utm_campaign=Technology+Review , accessed January 2018.
[37] Clustering is a machine-learning technique used to organize data into similar groups.
[38] Ibid.
[39] https://bootstraplabs.com/artificial-intelligence/applied-ai-conference-2016/ , accessed January 2018; one author [TAC] is a special advisor to BootstrapLabs Group, LLC.
[40] That we only use 10% of our brains is a myth – e.g., http://www.wired.com/2014/07/everything-you-need-to-know-about-the-10-brain-myth-explained-in-60-seconds/ , accessed January 2018. Even sleeping, we use most of our neurons to dream, breathe, digest food, beat our hearts, etc.
[41] P.A. Merolla, et al., “A million spiking-neuron integrated circuit with a scalable communication network and interface,” Science , 345 (6197), pp. 668-673, 8 August 2014.
[42] http://ieeexplore.ieee.org/document/880850/?reload=true , accessed January 2018.
[43] http://science.sciencemag.org/content/345/6197/668 , accessed January 2018.
[44] “The Josephson effect is the phenomenon of supercurrent—i.e., a current that flows indefinitely long without any voltage applied—across a device known as a Josephson junction (JJ), which consists of two superconductors coupled by a weak link.” https://en.wikipedia.org/wiki/Josephson_effect , accessed January 2018.
[45] https://phys.org/news/2018-01-nist-superconducting-synapse-piece-artificial.html , accessed January 2018.
[46] Complementary metal-oxide-semiconductor
[47] https://spectrum.ieee.org/nanoclast/semiconductors/devices/memristordriven-analog-compute-engine-would-use-chaos-to-compute-efficiently , accessed January 2018.
[48] https://newsroom.intel.com/editorials/intels-new-self-learning-chip-promises-accelerate-artificial-intelligence/ , accessed January 2018.
[49] https://www.engadget.com/2017/09/26/intel-loihi-neuromorphic-chip-human-brain/ , accessed January 2018.
[50] http://www.wired.co.uk/article/ai-neuromorphic-chips-brains , accessed January 2018.
[51] https://www.globalsecurity.org/military/library/budget/fy2016/army-peds/0601102a_1_pb_2016.pdf , accessed January 2018.
[52] https://inilabs.com/ , accessed January 2018.
[53] https://www.pegusapps.com/en/services , accessed January 2018.
[54] https://www.theverge.com/2018/1/27/16940002/google-clips-ai-camera-on-sale-today-waitlist ; https://www.prnewswire.com/news-releases/crowdflower-announces-third-wave-of-ai-for-everyone-challenge-winners-300587610.html , accessed January 2018.
[55] For example: “NVIDIA (NASDAQ:NVDA) stock has returned 80.1% so far in 2017, through Dec. 15, continuing its rapid rise that began last year, when the stock returned a whopping 227%.” [ https://www.fool.com/investing/2017/12/18/how-nvidia-crushed-it-in-2017-a-year-in-review.aspx , accessed January 2018.]. This growth is driven primarily by the intense demand for GPUs because of AI. We might anticipate similar stock price surges elsewhere as other advanced architectures come online for AI.
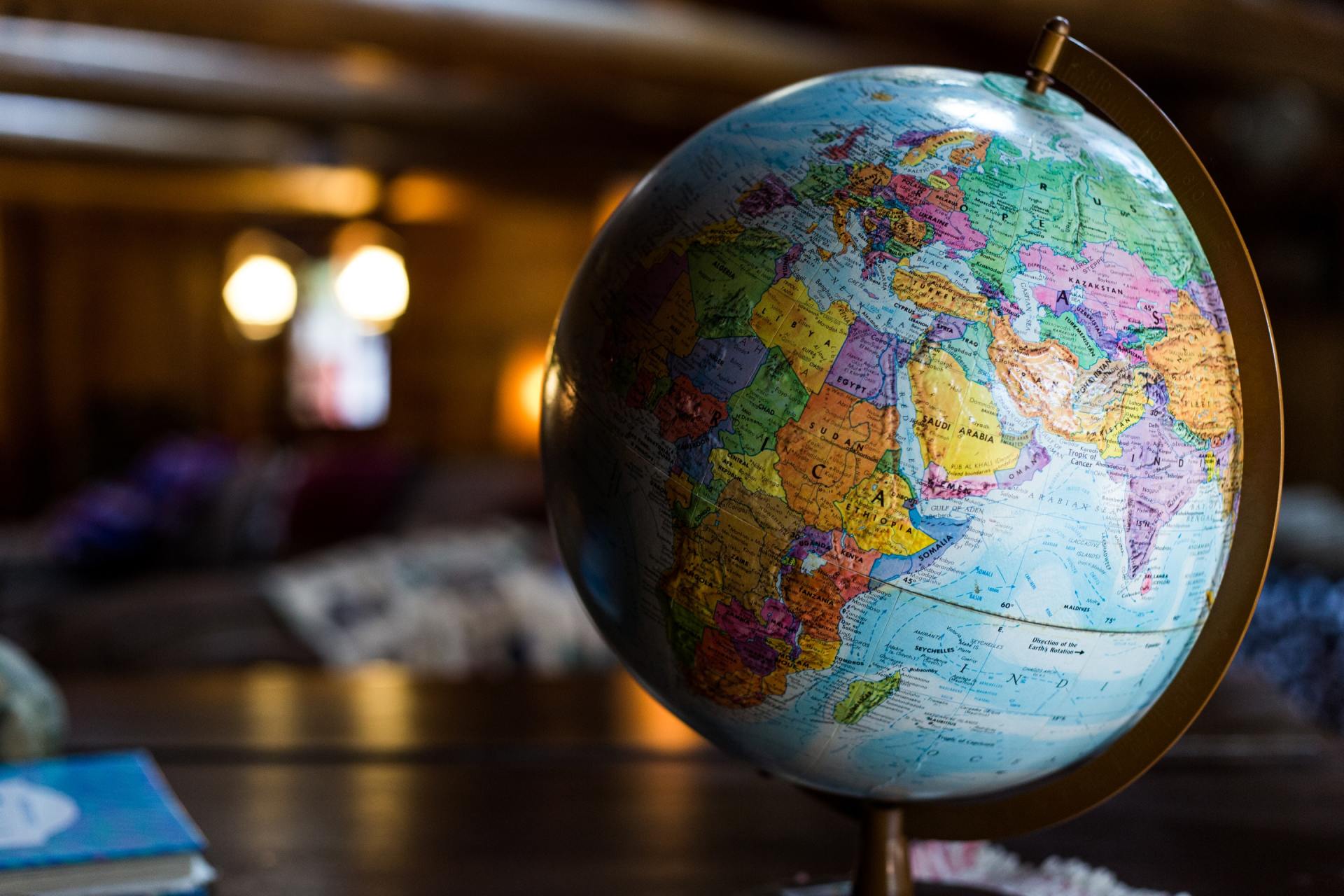
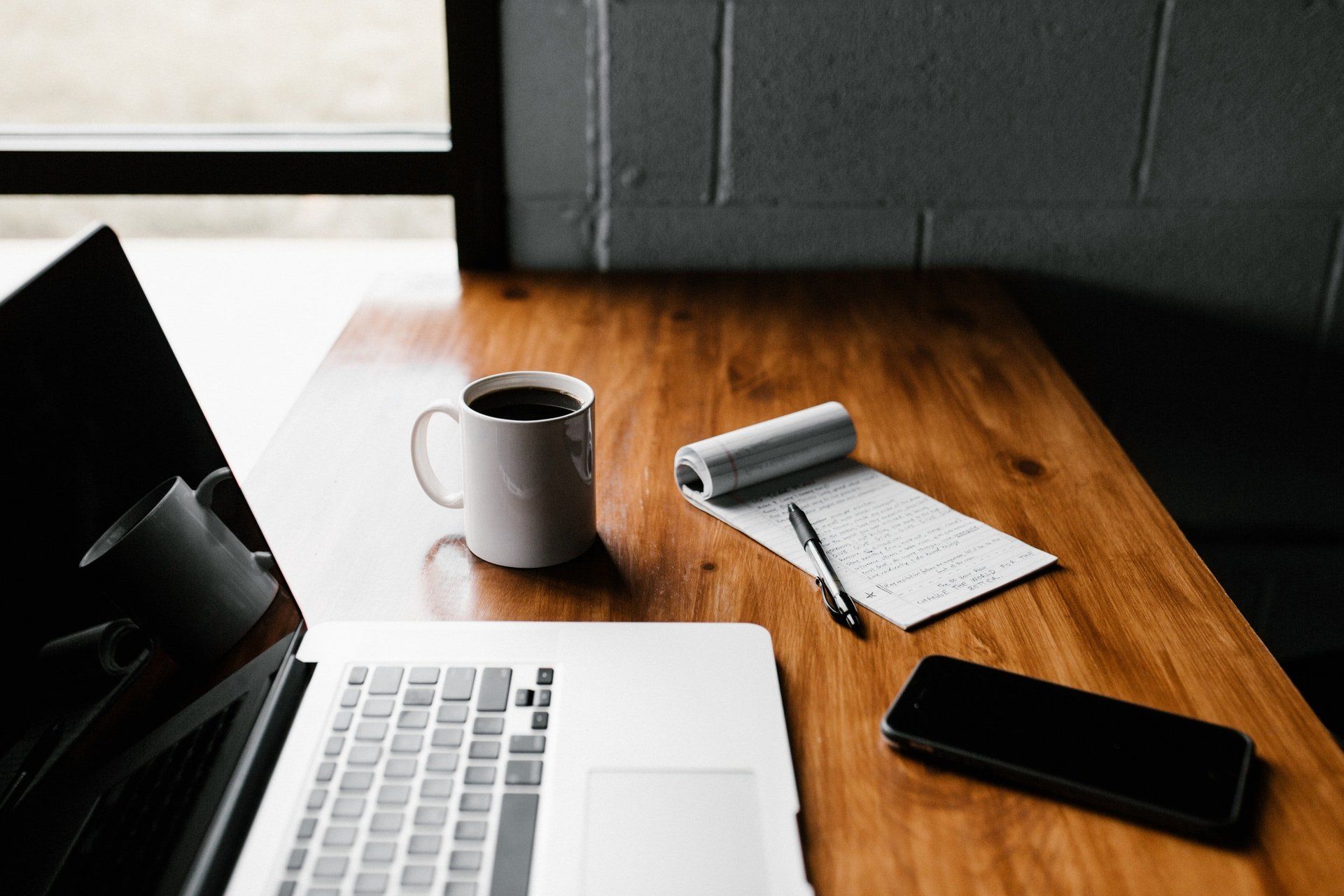
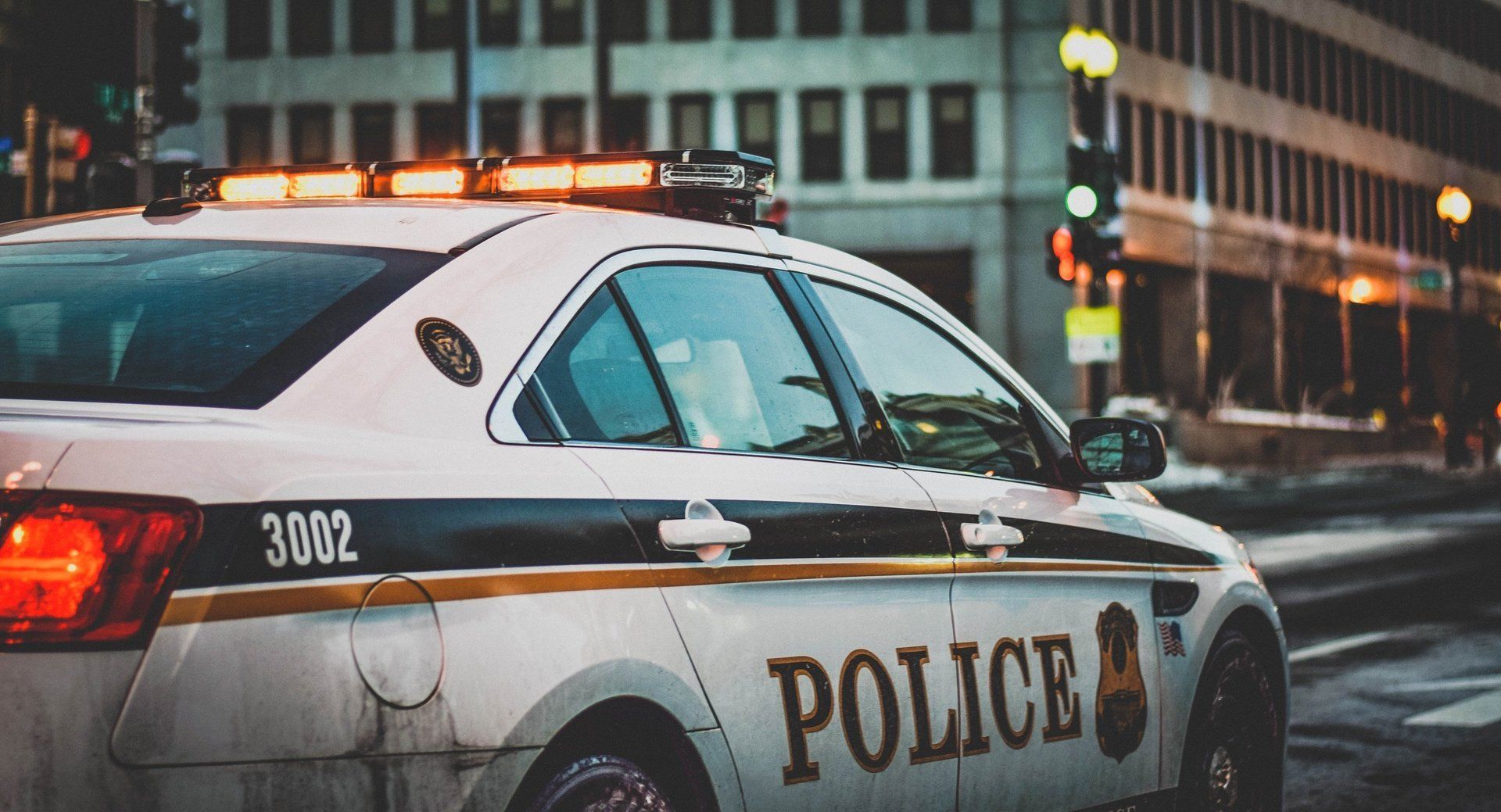
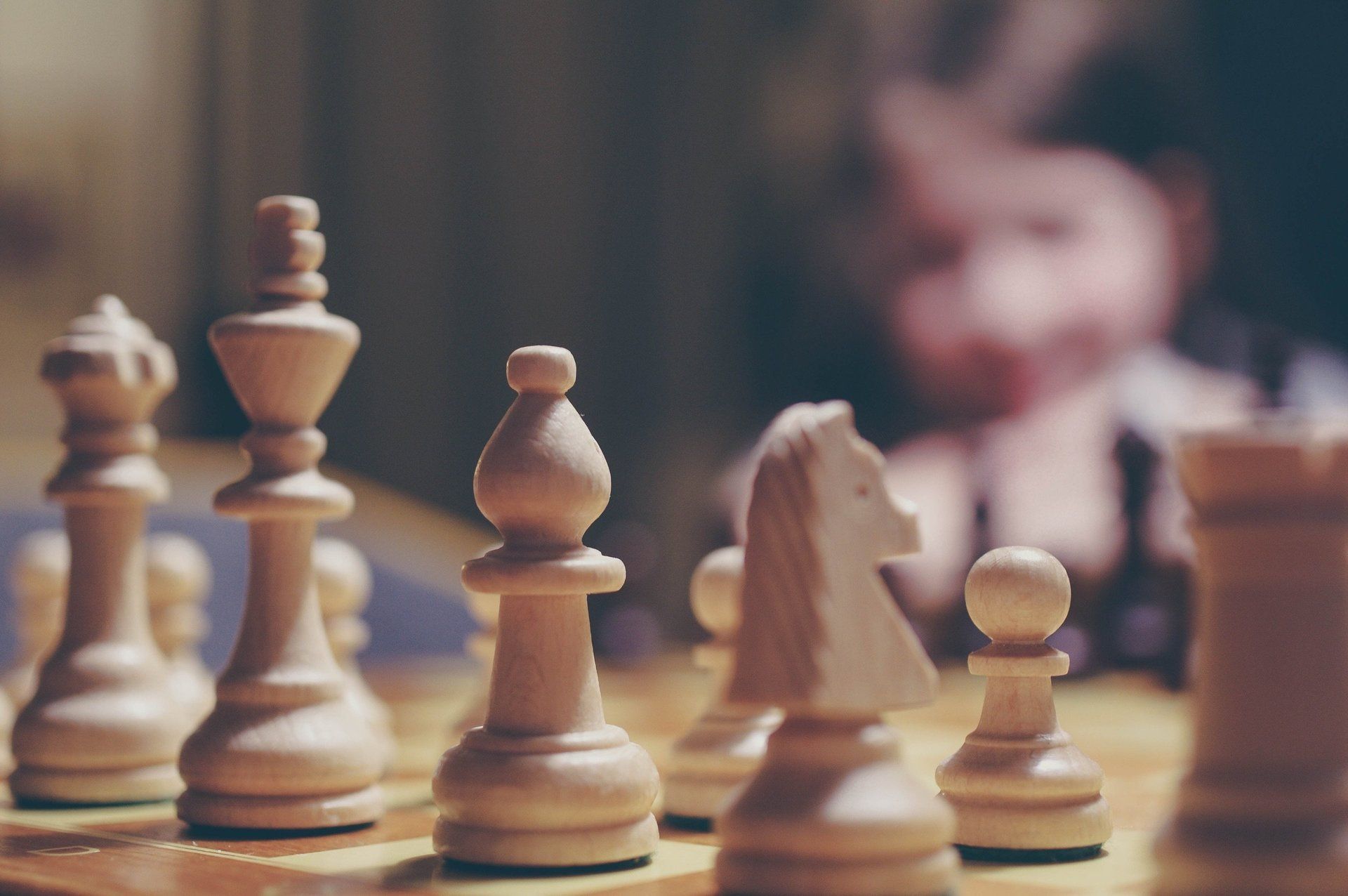